Tesla Insurance: Buffett Got It Wrong - 13 minutes read
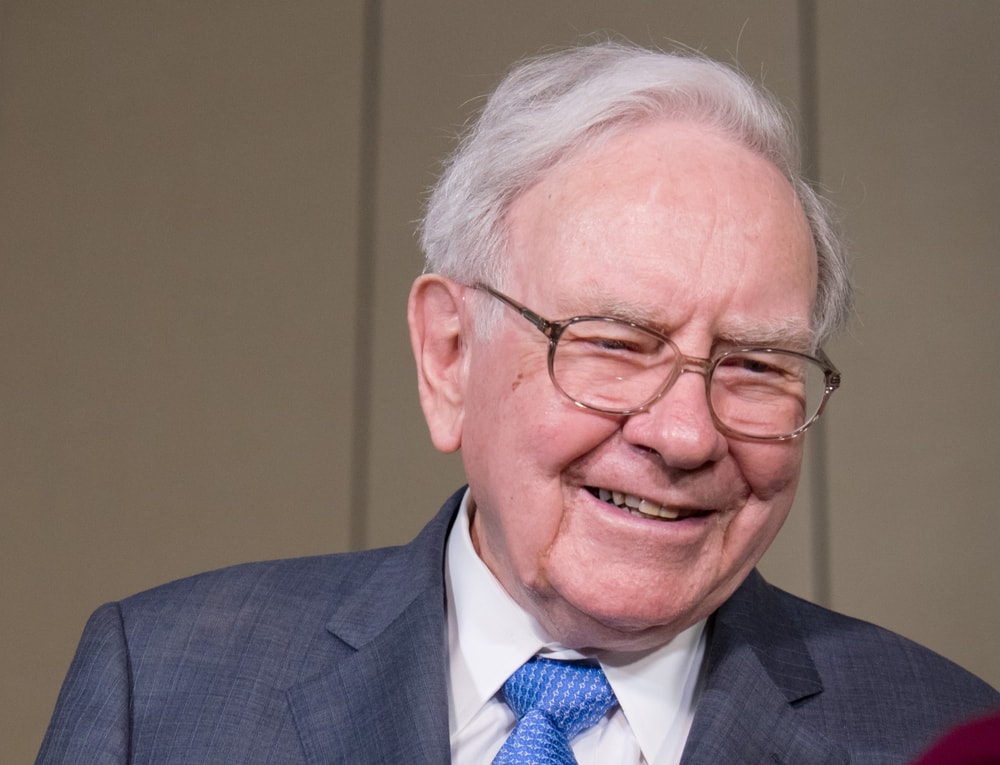
Telematics bring new data to light, which allow more accurate assessment of an individual's actual risk.
Car manufacturers have more and higher fidelity telematics data than insurers, allowing them to price individuals' premiums better.
Manufacturers will ultimately have all the low-risk drivers using their insurance offerings, and incumbents will have all the highest risk.
Warren Buffett, Chairman and CEO of Berkshire Hathaway (BRK.A) (BRK.B), of which GEICO is a wholly-owned subsidiary, recently claimed in response to Tesla's (TSLA) announcement of its planned insurance offering: "The success of the auto companies getting into the insurance business is probably as likely as the success of the insurance companies getting into the auto business."
It's my opinion that this statement is incorrect, as I'll explain below, first by showing how incumbent carriers' risk calculations are flawed, then explaining how vehicle manufacturers' risk models will likely work better, and finally demonstrating how that could change the entire industry.
Many people, when hearing that car manufacturers are planning to get into Insurance, instinctively respond with a knee-jerk reaction of "that's impossible". Insurance carriers have spent billions on infrastructure, regulatory approvals and actuarial risk assessment, and must maintain a boatload of cash to cover claims. That's all money that Tesla doesn't have available.
This is an incorrect belief. There exists in Insurance the concept of an MGA (or MGU), which is a type of agency allowed to brand itself as a carrier, leveraging a relationship with a "fronting carrier", which is an existing insurer on the back-end of the partnership. The MGA handles the pricing and underwriting (often with the fronting carrier's assistance), marketing, customer acquisition, and policy servicing, the fronting carrier handles the claims and money management, and they share the profits from the joint venture.
Many new carrier brands start out as MGAs. Two notable examples are:
Manufacturer-insurers will very likely follow this same model. In Tesla's case, they have already stated a partnership with Markel, a reinsurer, ostensibly as a fronting carrier. They have also stated the need to buy a shell, likely an existing MGA that's already licensed in many states. All that would leave is for them to build the software for customer acquisition and policy servicing. That should be a piece of cake for Tesla, which has a huge team of developers and a strong online presence already.
Now that the "it's impossible" myth is debunked, let's look at how car manufacturers are poised to outdo incumbent insurers at their own game.
Ratemaking for Auto Insurance traditionally utilizes Rating Tables for calculating a given insured party's risk relative to a Base Risk. A Base Risk is an arbitrarily selected profile defined by a carrier, usually to represent their typical exposure. For example, a carrier's Base Risk profile may look like this:
This Base Risk profile is priced for risk, generating a Base Rate. Each variable of the risk profile is then expanded into a rating table enumerating each potential value or value group, and assigning a rate relativity modifier or multiplier, based on the carrier's determination of how each value impacts risk.
As an example of how a Rating Table works, a slice of an age-and-gender collision rating table might look something like this:
And so on...
These Rate Relativity modifiers are then combined together (usually multiplied) to arrive at premiums for each type of coverage (collision, comprehensive, property damage, bodily injury, etc.). Those coverage-level premiums are then added together to arrive at a total premium, e.g.:
There are multiple factors that comprise an individual's risk profile, including:
Each of these factors' possible values is enumerated into a table, similar to the one I presented above, with modifiers for each value corresponding with the carrier's estimation of risk for that value.
This tabular method of ratemaking has been used for over 100 years.
The innate flaw in this type of ratemaking is in the same thing that makes it work: relative risk estimates are prospective and assessed in aggregate, not for individuals. For example, within the segment of 26-30 year-old males, per my sample table above, there's a 15% implied increased risk of a collision over the Base Risk, due to relative number of claims or driving anomalies the carrier has perceived in that age and gender category. However, this fails to account for the fact that some subset of the 26-30/m population yields a higher than average risk for the group, driving up the average risk of the entire group. Some 26-30 year-old men are exceptionally safe drivers, and pose a lesser risk than others who are exceptionally unsafe drivers. While other factors are considered in this exercise (e.g. ticket history may be used to distinguish safer individuals in this category), there is still a failure to look at each driver as an individual, due to low fidelity, or holes in the data, specifically regarding each actual driver's day-to-day driving behavior. Therefore, in the traditional rating model, for any given carrier, some drivers will overpay, while others will underpay for their actual risk.
Where this becomes a problem for carriers is in that no 2 carriers calculate rates in the same way. For various reasons, each carrier has developed its own rate modifiers for each variable value. So Carrier A may penalize a given age group more heavily while Carrier B may put more weight on ticket history in assessing risk. Therefore a 26-30 year-old male with no tickets might get a better rate with Carrier B, while one with tickets would stick with Carrier A.
Finally, the ability for drivers to self-select into the most beneficial carrier pricing model yields an inefficiency for carriers - each will ultimately wind up insuring a certain population of under-priced risk, yielding higher average loss ratios than necessary. High churn rates compound this problem, ironically, largely driven by carriers' own ads compelling drivers to shop around.
The world of Telematics for the first time in a long time brings new information into the world of auto insurance rating. By plugging a device in to their car's OBD port, or installing a smartphone app, drivers permit their insurance carriers access to information about their actual driving behaviors, which enables the carriers to assess risk more accurately per individual driver. Behaviors measured can include:
This is an improvement on the traditional rating model, because it offers carriers insight into actual driving behavior, where in the past, it could only be inferred from other data points like age, gender, and motor vehicle records.
However, even telematics systems have some flaws:
New vehicles from OEMs are coming equipped with very powerful, connected computer systems, which have peripheral input devices capable of sensing other vehicles and road conditions all around the car, GPS and mapping systems capable of inferring speed limits, sensors on the steering wheel and pedals, and more. Tesla (TSLA) is leading the pack on this front, with its Autopilot system, employing cameras, radar, and ultrasonic sensors, and with advanced processing power to analyze and identify risks that even humans often miss.
Driver-assist systems gather dozens of additional data-points beyond what a traditional telematics device is capable of. They can also collect actual driver behavior.
A couple years ago, I prototyped a basic telematics app using GM's (GM) Developer tools, and I was shocked at how much real-time driving data is available at every instant of a vehicle's operation.
With a stream-processing solution, car manufacturers will have the ability to analyze very precise driving behaviors, such as:
And so on...
More than any risk data currently analyzed in traditional ratemaking, these specific driving behaviors give much more specific insight into how each actual driver operates his or her vehicle, and how that behavior may increase or decrease the risk of an accident.
Thus, it follows that car makers' risk models will ultimately exceed the efficiency of traditional carriers' for rating risk for any specific individual.
The logical progression would drive one to believe that incumbents will follow OEMs by collecting telematics from on board systems. However, incumbents will have to ask drivers to install apps on their cars, adding friction.
In addition, historical driving data won't be immediately available to incumbents, so rates will have to be adjusted subsequently, after the data is collected. Without the data for retrospective analysis, incumbents' upfront quoted premiums can't be as attractive as possible at the time of purchase.
However, car manufacturers - especially those building FSD systems - will likely already have historical data, and will be able to simply ask permission to access the driver's previously anonymous records in the quoting process. In the case of Tesla, drivers already opt in to driving data collection, to help train AutoPilot. This same data could be used to calculate risk and generate a real-time rating of the user.
Manufacturers will have some additional benefits on their side, helping them compete with incumbents in other areas:
All around, the insured party's experience should be expected to be superior with an OEM insurer.
So what if the manufacturers can rate individuals better? Insurance works because risk is assessed in aggregate as a pool, so the total cost of claims for the whole will remain the same, right?
Well, yes... BUT...
When manufacturers are able to very accurately identify drivers who represent an anomalously low risk (e.g. a 20 year-old male who drives very safely), and will be able to provide those individuals lower rates to correspond to their actual individual risk, those anomalously low-risk drivers would probably migrate en masse to the manufacturers for cheaper, better insurance.
With a higher-risk population sticking with incumbent carriers, their loss ratios will rise and force them to increase rates across the board. As a result, this would probably push the middle tier of risk to manufacturers' insurance offerings as well, ultimately leaving incumbents only with the highest risk portion of the population.
With loss ratios for OEM-insured vehicles spiraling out of control, some large-scale incumbents may refuse to insure entire brands of vehicle, knowing that the risk is too severe. The remainder will migrate to non-standard carriers, who will be able to profit from the high-risk population (that's their specialty).
Unless traditional insurers find a way to collect and assimilate more accurate telematics data into their risk models, I don't see how they can compete with next-generation OEM-insurers.
This will be difficult, since manufacturers can collect that data innately, whereas incumbents will have to get app installs from drivers to get the same data (and again, the worst drivers probably won't opt in).
I only see two ways incumbents can compete with OEM insurers:
I don't see either of these scenarios as a near-term likelihood. While it's possible we could see this occur in the future, I would expect for the next several years that incumbents will be losing their best customers to OEMs' insurance offerings, and will wind up playing catch-up to try to get them back.
Thus, I believe Warren Buffett is wrong, and in fact has it backwards, when he suggests car makers can't be successful in insurance. I believe OEMs actually have a head-start. And unless they get moving on a telematics strategy ASAP, it's the incumbents who will be struggling to survive.
Disclosure: I am/we are long TSLA. I wrote this article myself, and it expresses my own opinions. I am not receiving compensation for it (other than from Seeking Alpha). I have no business relationship with any company whose stock is mentioned in this article.
Additional disclosure: DISCLOSURE: I AM NOT A FINANCIAL ANALYST OR ADVISOR. ALL CONTENT I CONTRIBUTE TO SEEKING ALPHA IS MY OPINION ONLY, AND DOES NOT CONSTITUTE ADVICE TO BUY OR SELL ANY SECURITIES. PLEASE DO YOUR OWN RESEARCH AND CONSULT A FINANCIAL PROFESSIONAL BEFORE INVESTING.
Source: Seekingalpha.com
Powered by NewsAPI.org
Keywords:
Tesla, Inc. • Insurance • Telematics • Data • Risk • Telematics • Insurance • Insurance • Risk • Insurance • Financial risk • Warren Buffett • Chairman • Chief executive officer • Berkshire Hathaway • Brka • GEICO • Subsidiary • Tesla, Inc. • Tesla, Inc. • Car • Insurance • Insurance • Insurance • Infrastructure • Regulation • Actuarial science • Risk management • Money • Insurance • Money • Tesla, Inc. • Belief • Insurance • Brand • Insurance • Partnership • Pricing • Underwriting • Marketing • Customer • Mergers and acquisitions • Insurance • Profit (accounting) • Joint venture • Brand • Manufacturing • Insurance • Tesla, Inc. • Partnership • Reinsurance • Royal Dutch Shell • A Piece of Cake • Tesla, Inc. • Insurance • Vehicle insurance • Insurance • Risk • Variable (mathematics) • Information theory • Special relativity • Multiplication • Risk • Theory of relativity • Insurance • Insurance • Insurance • Risk • Value (ethics) • Value (ethics) • Approximation • Value (ethics) • Table (information) • Scientific method • Nature (philosophy) • Type–token distinction • Object (philosophy) • Relative risk • Sampling (statistics) • Risk • Risk • Number • Gender • Fact • Subset • Statistical population • Risk • Social group • Risk • Lo-fi music • Risk • Problem solving • Risk • Old age • Risk • Shop Around • Telematics • Information technology • Vehicle insurance • Computer • Car • On-board diagnostics • Smartphone • Insurance • Risk assessment • Individual • Behavior • Measurement • Evaluation • Scientific modelling • Insight • Social influence • Behavior • Gender • Motor vehicle • Telematics • Original equipment manufacturer • Computer • Peripheral • Input device • Vehicle • Car • Global Positioning System • System • Sensor • Steering wheel • Tesla, Inc. • Tesla, Inc. • Front-wheel drive • Autopilot • System • Radar • Ultrasonic transducer • Advanced driver assistance systems • System • Telematics • Telematics • Mobile app • Real-time computing • Vehicle • Stream processing • Behavior • Risk • Data • Behavior • Vehicle • Behavior • Original equipment manufacturer • Telematics • Printed circuit board • System • Application software • Car • Friction • Data • Time • Full scale • Computer • Tesla, Inc. • Autopilot • Risk • Consumer • Manufacturing • Insurance • Original equipment manufacturer • Insurance • Sensitivity analysis • Manufacturing • Insurance • Risk • Risk • Risk • Insurance • Risk • Risk • Manufacturing • Insurance • Risk • Ratio • Original equipment manufacturer • Insurance • Vehicle • Economies of scale • Insurance • Vehicle • Risk • Insurance • Telematics • Data • Next-generation network • Original equipment manufacturer • Original equipment manufacturer • Original equipment manufacturer • Insurance • Warren Buffett • Insurance • Original equipment manufacturer • Head Start (program) • Telematics • ASAP (variety show) • Tesla, Inc. • Seeking Alpha • Company • Stock market • Corporation • Corporation • Financial analyst • Seeking Alpha • Opinion • Trade • Sales • Security (finance) • Research • Investment •