The Future of AI in Retail: Fueling Change for the Industry - 8 minutes read
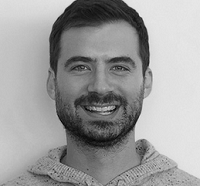
In this special guest feature, Matt Field, President and Co-Founder of MakerSights, says there’s no denying that AI and ML are starting to change the nature of the retail industry. One of its greatest benefits will be in how intelligent automation can be used by humans to augment their jobs. Drawing from his extensive background in both retail and technology as the former employee #1 at Birchbox, Matt is helping to transform the way brands develop and bring to market new products by combining voice of the customer software and predictive analytics. Through the MakerSights platform, leading apparel, accessories, footwear and home brands are able to de-risk their most important product decisions, making more of the products their customers want, and driving greater speed and profitability across their businesses.
Artificial intelligence (AI) and machine learning (ML) are no longer just buzzwords of tech giants and consulting firms. Many forward-thinking companies have also implemented AI and ML strategies to solve both new and existing business problems brought on by the deluge of data. The Retail industry is now getting in on the action. A recent global study found that two in five retailers and brands are already using intelligent automation such as AI and ML and adoption is expected to double by 2021. The study also found that intelligent automation is making the biggest impact in demand forecasting, supply chain planning and customer engagement.
In the last ten years, retail has jumped into the twenty-first century—high end, luxury fashion brands like Dior to more mainstream retailers like H&M are now collecting data about their customers’ preferences and applying it to their product development process. Prior to this, identifying which styles to produce and in what quantities were largely made based on gut instinct and historical sales data. While the fashion industry has led and defined style, it has lagged behind in applying technology like AI, ML and predictive analytics to their business operations. Poor forecasting has cost brands millions of dollars in unsold and marked down inventory, sometimes resulting in brands like Burberry and H&M burning merchandise rather than selling it for pennies on the dollar.
AI is now fueling change for the retail industry. Retailers like Target have started to recognize the benefits of a modern approach that leverages predictive analytics to best predict product demand and customer behavior. Others, such as Macy’s are using natural language processing techniques to fuel customer service chatbots. And some, including ASOS are leveraging AI for image analysis and visual search. For example, ASOS has deployed a tool that allows shoppers to upload a photo the shopper has taken—of an item a friend was wearing or something they saw in another store or from another source such as an image from the red carpet—to the brand’s app. The app then uses image recognition to search its catalog and return ASOS products to the shopper that most resemble those in the photo. Lululemon is using ML to collect and sort feedback by design, and then AI to optimize the supply chain and put new designs into production which resonate with consumers. With the “pull vs. push” model of gathering feedback through customer product reviews and pushing out newly generated products based on consumer preferences, Lululemon was able to determine which fabrics worked best for their designs and which ones missed the mark.
The Importance of Combining Human Intuition and Machine Learning
Despite the benefits of AI and ML, retailers have been challenged with adoption and implementation of these technologies within their organization; some, like many enterprises, have diminished the use of AI and ML to merely experimentation. According to Constellation Research, 92 percent of companies who say they’re currently using intelligent automation technologies (including AI, ML, deep learning, NLP and cognitive computing) report budgets of less than $5 million. In addition employees have resisted AI and ML in the workplace because of fear of losing their roles to automation. Another industry report also found that over a third retail product development professionals surveyed are concerned that adoption of new technologies may stifle creativity or replace humans in the workforce.
Companies like Zalando and Stitch Fix have successfully navigated this human-machine challenge. Instead of leveraging AI and automation in isolation to increase business efficiency, these companies are leaning into human-machine collaboration to drive even greater business results. Stitch Fix in particular has based their entire business model on data science, not only in recommendation systems, but also in human computation, resource optimization, inventory management, algorithmic fashion design and many others. The company uses explicit data and implicit data to predict what style to send to a client. Because customers enter sizing and style-preferences into the site before requesting a “fix”, Stitch Fix has a large amount of explicit data to consider, as their algorithms learn and track client preferences over time, both individually and as a whole. The company now employs hundreds of data scientists to help process and synthesize the massive amounts of data they collect from their subscribers to deliver product attributes that will resonate best with shoppers. This is done in combination with hundreds of remote human “stylists” and almost 3 million consumers manually entering answers to roughly 85 style-based questions into the service. The final step—what Stitch Fix notes as the most important part of its matchmaking process—incorporates quality control from the Stitch Fix stylist who also writes a personalized note to the subscriber before outfit selections are shipped out. This high level of personalization and human-machine collaboration is difficult for brick and mortar stores to mirror, and provides a distinct competitive advantage for Stitch Fix.
There’s no denying that AI and ML are starting to change the nature of the retail industry. One of its greatest benefits will be in how intelligent automation can be used by humans to augment their jobs. Embracing AI and ML and incorporating this with human intuition to predict, create and manage consumer preferences can help retailers increase their bottom line while meeting modern consumer expectations for personalized, on-trend and convenient products.
Sign up for the free insideBIGDATA newsletter.
Source: Insidebigdata.com
Powered by NewsAPI.org
Keywords:
Future • Artificial intelligence • Social change • Artificial intelligence • Nature • Welfare • Intelligence • Automation • Employment • Technology • Employment • Birchbox • Economic development • Product (business) • Voice of the customer • Software • Predictive analytics • Clothing • Footwear • Product (business) • Decision-making • Product (business) • Customer • Profit (accounting) • Business • Artificial intelligence • Artificial intelligence • Machine learning • Buzzword • Technology • Company • Artificial intelligence • Strategy • Business • Noah's Ark • Data • Retail • Globalization • Research • Artificial intelligence • Automation • Artificial intelligence • Education • Artificial intelligence • Automation • Demand forecasting • Supply chain management • Customer engagement • Retail • Luxury goods • Luxury goods • Christian Dior SE • Retail • H&M • Data • New product development • Quantity • Intuition • History • Sales • Data • Design • Technology • Artificial intelligence • Predictive analytics • Business operations • Forecasting • Inventory • Burberry • H&M • Product (business) • United States dollar • Retail • Retail • Target Corporation • Employee benefits • Predictive analytics • Product (business) • Consumer behaviour • Macy's • Natural language processing • Customer service • Chatbot • ASOS.com • Artificial intelligence • Image analysis • Visual search • ASOS.com • Brand • Mobile app • Mobile app • Computer vision • ASOS.com • Product (business) • Lululemon Athletica • Artificial intelligence • Supply chain • Manufacturing • Consumer • Feedback • Customer • Product (business) • Consumer • Lululemon Athletica • Intuition • Machine learning • Welfare • Artificial intelligence • Maximum likelihood estimation • Technology • Organization • Business • Artificial intelligence • Maximum likelihood estimation • Experiment • Constellation • Research • Intelligence • Automation • Technology • Artificial intelligence • Maximum likelihood estimation • Deep learning • Natural language processing • Cognitive computing • Employment • Artificial intelligence • Employment • Automation • Industry • Retail • New product development • Emerging technologies • Creativity • Company • Zalando • Stitch Fix • Artificial intelligence • Collaboration • Stitch Fix • Data science • Recommender system • Human-based computation • Mathematical optimization • Stock management • Algorithm • Fashion design • Company • Data • Data • Customer • Customer • Preference • Stitch Fix • Algorithm • Machine learning • Consumer • Preference • Holism • Scientific method • Stitch Fix • Quality control • Stitch Fix • Automotive design • Personalization • Personalization • Collaboration • Brick and mortar • Website • Competitive advantage • Stitch Fix • Artificial intelligence • Nature • Welfare • Intelligence • Automation • Human • Employment • Artificial intelligence • Corporation • Human • Intuition • Consumer • Retail • Consumer • Product (business) •