How Is Machine Learning Transforming Small Business Lending? - 6 minutes read
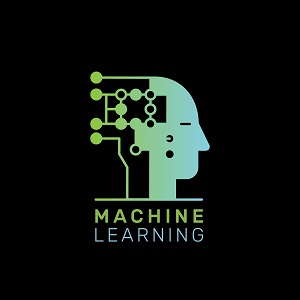
Small and medium-sized businesses are the keystone of the modern-day labor market. In the United States alone, small businesses employ almost 50% of the private workforce, and recent data shows that companies with fewer than 20 employees have added 1.2 million net new jobs.
But although their growth is vital to a sustainable global economy, SMBs continue to struggle to get the funding they need. The traditional lending system simply isn’t set up to meet the smaller capital needs of these types of enterprises: taking into account the risks and the long review process, small business loans typically don’t pay off for banks. Chances of being accepted are incredibly low for businesses that aren’t already well-established, and they rarely have the structure to carry them through the long review process anyway.
It has been an incredibly frustrating road for entrepreneurs, but thanks to developments in AI and data science, the alternative lending market has opened the doors to new opportunities. Here’s how digital lenders are utilizing machine learning to reshape the industry and provide a growing number of small enterprises with the capital they need.
We’re witnessing how machine learning is improving fraud prevention across all industries and platforms, and it’s no different with the financial sector. When it comes to SMB lending, where fraud detection is a critical concern of the underwriting process, predictive models are being trained to assess risks by analyzing cases of financial fraud. To determine the risks based on application characteristics, the models then analyze data such as:
The model then presents the results as a percentage probability of fraud. This way of assessing risk is not only significantly more informed, but it also helps speed up the process as underwriters can immediately know which applications to flag.
In the traditional lending system, applicants are reviewed for creditworthiness by examining a very narrow range of data. It’s clear that in today’s marketplace, credit history and Paydex scores don’t paint a clear picture of a firm’s potential. And when it comes to newer businesses looking for a loan, their chances are instantly trumped by the notorious issue of “insufficient credit history”.
In the digital lending market, machine learning models are being used to present exponentially larger sets of data and scenarios that will help paint a much more detailed picture of the applicant’s creditworthiness. Lenders can review real-time enhanced data that reflects the health and potential of a business, such as customer reviews and real-time shipping trends. As a result, loan applicants are reviewed more fairly while lenders are able to create advanced risk profiles.
Improving the Efficiency of the Underwriting Process
Underwriting automation is helping overcome the second major obstacle in small business lending: the length of the entire process.
Most small businesses don’t have the capital to carry them through the review process, which takes months before lenders can determine financial eligibility and set the pricing model. In the meantime, lenders have to collect data from various sources, clean the data sets, and extract the necessary information. In practice, they also have to get back to the applicant several times throughout the process to collect missing information, often ending up with inconsistent data which further prolongs the work.
All of this is solved by establishing predictive models to streamline the underwriting process. For example, a model can be trained to calculate the probability of a loan defaulting within a certain period. This prediction will be used to assess the applicant’s financial eligibility and set the pricing. It’s a win-win situation for both parties, as businesses will gain access to capital much faster, while lenders can optimize their workflows and significantly improve profitability.
Machine learning is transforming small business lending beyond the underwriting process. Once a loan is approved, lenders have to track an expanding amount of information as part of on-going loan monitoring. Using machine learning, they’re able to rely on an efficient loan-monitoring system to track and analyze tremendous amounts of customer data. With this advanced system in place, they’re able to pinpoint any potential problems at their earliest stages and take action before the borrower defaults. Effective loan monitoring is helping make SMB lending less risky and more profitable.
Without a doubt, machine learning is profoundly transforming SMB lending, at the benefit of both lenders and borrowers. Through predictive models, data analysis, and effective loan monitoring, we’re seeing new solutions to notorious issues of the lending industry. We have yet to see the technology fully implemented in the traditional lending system, but we’re already witnessing a new era of the financial industry.
Natasha Lane is a lady of a keyboard with a rich history of working in the IT and digital marketing fields. She is always happy to collaborate with awesome blogs and share her knowledge all around the web. Besides content creating, Natasha is nowadays quite passionate about helping small business to grow strong.
Sign up for the free insideBIGDATA newsletter.
Source: Insidebigdata.com
Powered by NewsAPI.org
Keywords:
Machine learning • Day labor • Labour economics • United States • Small business • Private sector • Workforce • Data • Company • Employment • Internet • Employment • Economic growth • Sustainability • World economy • Small and medium-sized enterprises • Funding • Loan • System • Capital (economics) • Business • Deposit account • Risk • Small business • Systems engineering • Artificial intelligence • Data science • Market (economics) • Machine learning • Capital (economics) • Machine learning • Fraud • Financial services • Small and medium-sized enterprises • Loan • Fraud • Underwriting • Business process • Predictive modelling • Risk management • Analysis • Case study • Risk • Software • Data analysis • Mathematical model • Probability • Risk • Business process • Underwriting • Application software • Loan • System • Credit risk • Data • Credit history • Paydex • Credit history • Digital data • Marketing • Machine learning • Mathematical model • Data • Image • Credit risk • Real-time computing • Data • Business • Customer • Loan • Underwriting • Underwriting • Automation • Small business • Loan • Capital (economics) • Business process • Finance • Conceptual model • Information • Underwriting • Probability • Loan • Finance • Capital (economics) • Workflow • Profit (accounting) • Machine learning • Small business • Loan • Underwriting • Business process • Loan • Loan • Information • Loan • Machine learning • System • Customer • Data • System • Debtor • Default (finance) • Loan • Small and medium-sized enterprises • Loan • Risk • Profit (economics) • Machine learning • Small and medium-sized enterprises • Loan • Employee benefits • Loan • Debtor • Predictive modelling • Data analysis • Loan • Technology • Computer keyboard • Digital marketing • Blog • Knowledge • World Wide Web • Small business •